Are you looking to elevate your AI-driven projects with advanced techniques in human pose estimation or seeking to bring unparalleled realism to augmented reality experiences? If so, understanding and utilizing skeleton annotation could be your gateway to success. In an industry projected to soar beyond $48 billion by 2023, the precision and depth offered by skeleton annotation are not just advantageous, they're essential.
Skeleton annotation is more than a tool, it's a transformative approach to computer vision, enabling the detailed mapping and analysis of movement. This technique is pivotal in various high-stakes applications, from enhancing sports analytics with intricate motion tracking to revolutionizing animation with lifelike fluidity. It's not just about plotting points, it's about connecting them to reveal the complexity of motion and structure in a way that wasn't possible before.
However, venturing into skeleton annotation without a solid understanding of its principles and potential applications can be overwhelming. This guide is crafted to navigate you through the nuances of skeleton annotation. We'll explore its fundamental concepts, diverse applications, and why it's becoming indispensable in training cutting-edge computer vision models. With the right knowledge, you can harness the full power of skeleton annotation, ensuring your projects not only meet but exceed the demands of an ever-advancing technological landscape.
What is the Skeleton Annotation
Skeleton annotation in the realm of computer vision, particularly in human pose estimation, is a process that involves the identification and mapping of key points on the human body, typically at joint locations such as elbows, knees, shoulders, and hips. These points are then connected to form a skeletal structure, essentially creating a virtual 'skeleton' that represents the interconnected nature of human anatomy in a digital format. This method offers a more structured and detailed means of analyzing the human form and its movements, surpassing traditional methods that focus on external contours or isolated body parts.
The evolution of skeleton annotation is closely tied to the advancements in computer vision and machine learning. Initially, the techniques were rudimentary, primarily focusing on basic joint detection. However, the introduction and progression of deep learning technologies have significantly enhanced the accuracy and sophistication of these methods. The importance of skeleton annotation in computer vision cannot be overstated, it provides a comprehensive framework for understanding and analyzing human posture and movement in a nuanced manner. This technology has revolutionized human pose estimation, enabling precise real-time tracking and analysis of human motion. Its applications are diverse, ranging from augmented reality and animation to sports analytics and physical therapy, illustrating a significant leap in the capabilities of computer vision systems. Skeleton annotation allows computers to understand and analyze human motion, paving the way for more natural and intuitive human-computer interactions.

Advanced Technology Behind Skeleton Annotation
The advent of machine learning, especially deep learning, has been a game-changer in the field of skeleton annotation. At the heart of these technologies are sophisticated algorithms like Convolutional Neural Networks (CNNs) and Recurrent Neural Networks (RNNs), which have revolutionized the way computers interpret visual data. CNNs are particularly effective in image recognition tasks, making them ideal for identifying key points on the human body in static images. Meanwhile, RNNs excel in handling sequential data, which is crucial for analyzing video frames to track and understand dynamic human movements over time. By training these neural networks on extensive datasets of annotated images and videos, they learn to accurately predict the locations of key body joints and how they connect to form the human skeletal structure.
Computer vision plays a pivotal role in skeleton annotation, employing a suite of techniques for keypoint detection and pose estimation. Keypoint detection involves identifying significant points of interest in the body, such as joints and extremities. This process utilizes various computer vision methods including edge detection, shape recognition, and feature extraction. These methods enable the precise localization of keypoints, which are critical for constructing the skeletal framework. Following keypoint detection, pose estimation algorithms come into play, piecing together the positions and orientations of various body parts. This step is crucial in assembling a coherent skeletal structure, providing a clear depiction of the human posture and movement in both two-dimensional and three-dimensional spaces.
Skeleton annotation technology has found its way into numerous advanced applications, transcending the realms of basic image and video analysis. In more sophisticated implementations, 2D skeletal data is often used to reconstruct 3D models of the human body, providing a comprehensive view of movement and posture in real-world scenarios. This is particularly beneficial in fields such as augmented reality, animation, and biomechanics. Furthermore, the integration of skeleton annotation with real-time processing capabilities has opened up new avenues in interactive applications, including sports analytics, physical therapy, and human-computer interaction. The ability to process and analyze skeletal data in real-time requires optimized algorithms and often the utilization of high-performance computing resources like GPUs, ensuring swift and accurate skeletal tracking and analysis.
Keypoint vs. Skeleton: Decoding their Roles in Computer Vision
To fully appreciate the advancements in human pose estimation technology, it's crucial to understand the differences between skeleton and keypoint annotations. These two methods, while related, serve distinct purposes and offer different levels of insight into computer vision applications.
Keypoint annotation and skeleton annotation differ fundamentally in their approach and application. Keypoint annotation involves marking specific points on an image, typically identifying crucial anatomical landmarks such as joints on a human figure. In contrast, skeleton annotation builds upon keypoint data by connecting these points, forming a representation of the skeletal structure. This connection of points enables skeleton annotation to provide a visual representation of how different body parts are linked, something keypoint annotation does not inherently offer. Practically, this means that skeleton annotation can reveal insights into the dynamics and mechanics of human movement that are not apparent through keypoint annotation alone, due to its ability to portray the body as an integrated, cohesive system.
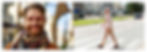
The advantage of skeleton annotation lies in its comprehensive portrayal of human movement and structure. Labeling the connections between key points facilitates a deeper analysis of human posture, alignment, and the biomechanics of movement. This level of detail is particularly vital in fields such as sports analytics, biomechanics, and physical therapy, where understanding the interaction between different body parts is crucial. Additionally, in the domains of animation and augmented reality, skeleton annotation enables the creation of movements that are more realistic and true to the natural mechanics of the human body. This comprehensive nature of skeleton annotation underscores its value in scenarios where a full understanding of human movement and posture is essential.
Skeleton Annotation: Diverse Applications in a Digital World
Skeleton annotation, a sophisticated extension of keypoint annotation, finds its applications in numerous domains, each leveraging its ability to provide a detailed understanding of movement and structure. Its usage goes beyond simple point marking, offering insights into the interconnected nature of body parts. This technique is particularly vital in areas where the relationship and dynamics between different body parts are crucial.
Human Pose Estimation in Advanced Applications
One of the primary uses of skeleton annotation is in advanced human pose estimation. This is crucial in fields like sports analytics, where it provides a detailed analysis of athletes' movements, aiding in performance optimization and injury prevention. It's also extensively used in biomechanics for analyzing human motion, which is essential in developing better ergonomic solutions and rehabilitation techniques.

Enhancing Realism in Animation and Virtual Reality
In the entertainment industry, especially in animation and virtual reality, skeleton annotation is key to creating realistic and complex character movements. By understanding how different parts of the body are interconnected, animators can create more lifelike characters and movements, significantly enhancing the viewer's experience. This application extends to virtual reality, where accurate skeletal tracking ensures immersive and interactive experiences.
Healthcare and Physical Therapy
Skeleton annotation plays a significant role in healthcare, particularly in physical therapy and ergonomics. By analyzing how patients move and hold their bodies, therapists can develop more effective treatment plans. It also aids in the design of ergonomic products by providing insights into natural body movements and postures.
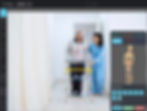
Robotics and Humanoid Robots
In robotics, especially in the development of humanoid robots, skeleton annotation aids in programming robots to mimic human movements more naturally. This is essential in applications where robots interact with humans or perform tasks requiring human-like dexterity.
Motion Capture and Interactive Systems
Skeleton annotation is instrumental in motion capture technology, used in both gaming and film production. It enables accurate tracking of actors' movements, which are then translated into digital models. In interactive systems, such as advanced gesture recognition interfaces, skeleton annotation facilitates more nuanced and responsive interactions.
How to Use Skeleton Annotation with BasicAI: A Step-by-Step Guide
Step 1: Data Upload
Starting with Data Selection: The first step in using BasicAI Cloud* for skeleton annotation involves selecting the data you wish to annotate. This platform is versatile, supporting various data forms including local files, URLs, and cloud storage. For instance, if you are aiming to annotate the pose of a ski player, you would begin by uploading an image of the ski player to BasicAI Cloud*. The process is straightforward: select your image and click the upload button.

Step 2: Setting the Ontology
Once your data is successfully uploaded, the next step is to set the ontology, which is essential for categorizing and defining the data for annotation.
Creating and Customizing Ontology: Click the "create" button to start setting up your ontology. Fill in the necessary information to tailor the ontology to your project. For example, in annotating a ski player, you would name the ontology 'ski', choose red as the label color, and select 'skeleton' as the tool type.

Defining Points for Annotation: The platform may provide a reference image showing the points to be annotated. You can adjust these points according to your project's specific requirements. Once you have configured these settings, your ontology setup is complete.
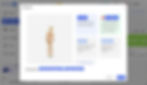
Step 3: Annotating Data with Skeleton Tools
Navigating to the Annotation Interface: Now, proceed to the annotation interface. Here, you'll select the skeleton tool. A supplementary interface will appear, typically on the left side, to guide you through the annotation process.
Performing Precise Annotation: Follow the instructions on the small interface to annotate your data accurately. This tool is designed to assist in precise placement and connection of key points, ensuring your skeleton annotation is as accurate as possible.
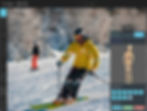
Saving Your Work: After completing the annotation, don’t forget to save your work. Click the 'save' button to ensure all your labeled data is securely stored.
Takeaways
As we wrap up our journey through the intricacies of skeleton annotation with BasicAI, it's evident that BasicAI offers more than just a toolset. It's a comprehensive ecosystem for computer vision advancements. BasicAI distinguishes itself by providing not only a robust platform for data annotation but also a suite of professional data annotation services. This dual capability empowers computer vision engineers to not only navigate the complexities of skeleton annotation with ease and precision but also to leverage expert services for large-scale, sophisticated projects. Whether it's the hands-on task of annotating data or the need for expertly curated datasets, BasicAI stands as a versatile and indispensable ally in the ever-evolving field of computer vision. As the demand for detailed and accurate visual data interpretation grows, BasicAI's combination of cutting-edge tools and professional services positions it as an essential resource for innovators and practitioners aiming to redefine the boundaries of what's possible in image and video analysis.
* To further enhance data security, we discontinue the Cloud version of our data annotation platform since 31st October 2024. Please contact us for a customized private deployment plan that meets your data annotation goals while prioritizing data security.
Read Next
Bounding Box Annotation: Insightful Tips, Case Studies, and Best Practice
Unraveling the Mysteries of Polygon Annotation: A Deep Dive into the World of AI with BasicAI
Image Segmentation: 10 Concepts, 5 Use Cases and a Hands-on Guide [Updated 2023]
Annotate Smarter | 2D & 3D Sensor Fusion Data Segmentation Guide [2023]
Annotate Smarter | Sensor Fusion Data Annotation: Manual Work Can Also Be Stress-Free
Annotate Smarter | How to Annotate 3D LiDAR Point Cloud 82 Times Faster with Higher Accuracy?
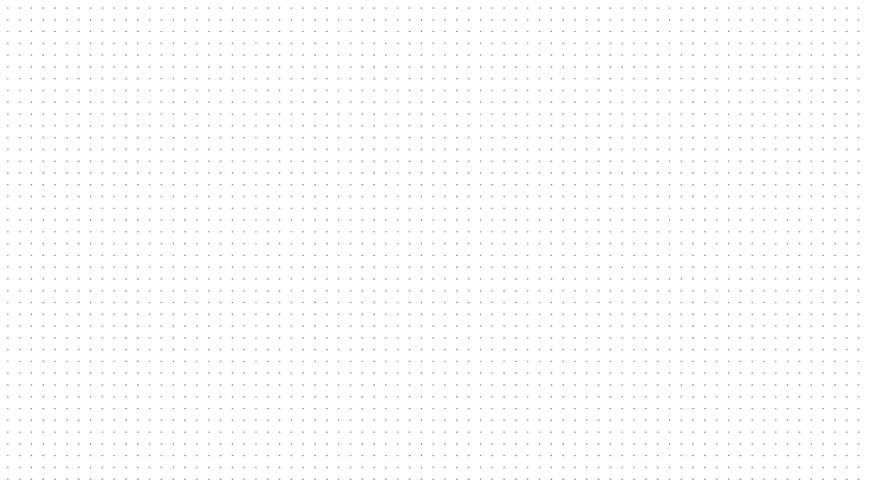