We have discontinued our cloud-based data annotation platform since Oct 31st. Contact us for private deployment options.
Precision is paramount in architecture and engineering. To achieve the highest levels of accuracy, the industry is increasingly turning to AI technology. However, from microscopic architectural details to macroscopic urban planning, these AI models rely on highly accurate data for their training.
LiDAR technology has emerged as a common tool, offering unprecedented 3D insights. Yet, merely collecting LiDAR data is far from sufficient, the true power of this technology lies in effective data annotation.
High-quality annotated LiDAR data holds significant potential for improving project design accuracy and reducing errors. Through professional annotation, raw point cloud data can be transformed into actionable intelligence, enabling teams to make more informed decisions and advance projects with greater confidence.
To delve deeper into the importance of LiDAR annotation, this article will explore the following key areas:
LiDAR Annotation: The Key to Next-Generation Architecture and Engineering
High-Quality LiDAR Annotation: Driving AI Innovation in Architecture and Engineering
BasicAI Annotation Services: Breaking Through Bottlenecks, Driving Project Success
LiDAR Annotation: The Key to Next-Generation Architecture and Engineering
LiDAR (Light Detection and Ranging) technology provides high-precision 3D spatial data for the architecture and engineering sectors by emitting laser pulses and measuring their return time. However, this raw point cloud data is not directly usable in its original form. LiDAR annotation is the crucial process that transforms this raw data into meaningful information. Through annotation, we identify and classify various elements within the point cloud, such as building structures, terrain features, and infrastructure components, converting abstract point sets into comprehensible and actionable 3D models. This process not only enhances data interpretability but also significantly increases its practical value across various applications.
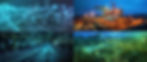
Key applications of LiDAR annotation in architecture and engineering include:
Building Information Modeling (BIM) Enhancement: LiDAR data annotation helps AI models enable precise identification of building components such as walls, doors, windows, and piping, creating more accurate digital twin models. This allows architects and engineers to conduct more precise structural analysis and performance simulations.
Urban Planning Optimization: AI models trained on well-annotated LiDAR data can accurately distinguish urban elements like buildings, roads, and green spaces, providing urban planners with detailed 3D city models. This aids in better land use planning and public space optimization.
Cultural Heritage Digitization: By processing finely annotated LiDAR scans of historical buildings, advanced computational techniques produce highly detailed digital archives, aiding in preservation and restoration efforts.
Intelligent Infrastructure Management: Remote monitoring of facility conditions and timely identification of maintenance needs are made possible through AI-powered analysis of annotated LiDAR data, which enables precise recognition of various infrastructure components.
Smart Structural Health Monitoring: By analyzing LiDAR data from different time periods using advanced comparison algorithms trained on annotated datasets, minute deformations in buildings or bridges can be accurately detected, providing critical information for structural health assessments.
High-Quality LiDAR Annotation: Driving AI Innovation in Architecture and Engineering
High-quality LiDAR annotation data acts as a "super nutrient" for AI models, significantly enhancing their performance in the field of architecture and engineering. Imagine if ordinary 3D scan data were the "eyes" of AI; then meticulously annotated LiDAR data would be like fitting these eyes with high-definition, ultra-wide-angle "smart lenses". With these "smart lenses", AI can not only see more clearly but also understand every detail it perceives.
This high-quality data markedly improves the accuracy and functionality of building models in multiple aspects. When training Building Information Models (BIM), precise annotation data enables AI models to accurately identify building components and features within point clouds, achieving automated information modeling. Moreover, these models can play crucial roles in construction site safety monitoring, structural health analysis, precise spatial planning, and indoor navigation.
For instance, in analyzing the architecture and landscape of traditional villages, AI models trained on high-quality LiDAR annotation data have achieved high accuracy rates of 81% and 83% in identifying buildings and vegetation, respectively. This high-precision recognition capability provides critical support for comprehensive analysis and understanding of the characteristics of traditional villages like Taoping Village, laying a solid foundation for cultural heritage preservation efforts.
As the quality of LiDAR data annotation continues to improve, the application scope of AI models is expanding, gradually encompassing more complex scenarios. McKinsey Global Institute's report predicts that by 2035, digital technologies, including AI, could increase productivity in the construction industry by up to 15% and reduce costs by up to 6%. This forecast highlights the importance of high-quality LiDAR annotation data for AI models and the enormous potential they collectively bring to architecture, engineering, and related construction activities.
The Five Major Hurdles in LiDAR Data Annotation
While LiDAR technology holds immense promise in architecture and engineering, the process of data annotation still faces significant hurdles.
Foremost among these is the sheer complexity and volume of data. LiDAR scans typically generate millions of point cloud data points, requiring annotators to precisely define boundaries, identify features, and categorize various elements within this dense information. This task is further complicated by the need to consider multiple dimensions, including spatial positioning, reflectivity, and color data, significantly increasing the complexity of annotation.
The demand for precision and standardization presents another formidable challenge. In architecture and engineering, even minor inaccuracies can lead to major issues in the design and execution phases. Consequently, annotations must maintain extreme accuracy from overall structures down to the finest details, while also adhering to strict industry standards. This challenge extends to maintaining consistent annotation standards across different projects and integrating LiDAR data with other sources such as photographs and CAD drawings.
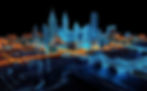
Resource intensity and technological adaptation pose additional significant challenges. The LiDAR data annotation process is not only time-consuming and labor-intensive but also requires deep domain knowledge and advanced data processing skills. As LiDAR technology rapidly evolves, annotation teams must continually update their methods and tools to adapt to new data formats and characteristics. This includes effectively integrating emerging technologies like AI and machine learning into annotation workflows and striking a balance between automation and human verification.
Environmental complexity and dynamic changes further complicate annotation efforts. Urban settings, with their intricate mix of buildings, infrastructure, and natural elements, increase annotation complexity. Historic or unique architectural structures may defy standard categorization. Moreover, capturing dynamic changes in building environments, such as ongoing construction processes or seasonal variations, adds another layer of difficulty to annotation work.
Lastly, privacy, security, and data management concerns cannot be overlooked. Detailed LiDAR data of buildings and infrastructure often contains sensitive information, necessitating a delicate balance between data utility and protection. This challenge extends to managing and updating large volumes of annotated data throughout project lifecycles, especially when dealing with both public and private spaces.
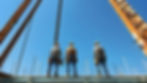
In light of these challenges, the architecture and engineering industry is exploring advanced LiDAR data annotation solutions. Effective solutions should be capable of processing massive datasets, ensuring high accuracy and consistency, adapting to various environments, and maintaining data security. This requires a combination of advanced technology, rich experience, and specialized teams. As technology progresses and industry standards evolve, new methodologies are emerging, opening up possibilities for enhanced LiDAR applications in architecture and engineering. These advancements aim to improve project efficiency and offer deeper insights, potentially transforming how the industry utilizes 3D point cloud data.
BasicAI Annotation Services: Breaking Through Bottlenecks, Driving Project Success
Building on these industry needs, BasicAI delivers comprehensive LiDAR annotation services that address the complex challenges of the AEC sector. The company specializes in transforming intricate LiDAR data into actionable insights, enabling more informed decision-making throughout project lifecycles.
BasicAI's core strength lies in its expert team, which brings extensive experience in 3D point cloud annotation for AEC projects, ensuring high-quality results that meet industry standards. The team excels in handling project data ranging from interior renovations to complex infrastructure developments. This expertise ensures precise annotation of building facade details, MEP systems, and specialized engineering environments.
To enhance efficiency and accuracy, BasicAI employs advanced AI-assisted tools that swiftly process large volumes of building scan data while ensuring geometric precision. These tools support a variety of annotation methods, including bounding boxes, polygons, and segmentation.
BasicAI's services are highly adaptable, offering tailored solutions based on project scale and requirements. Quality control is at the heart of the company's process, with each project undergoing multiple reviews to meet industry standards and client needs.
Through these comprehensive professional services, BasicAI is committed to driving innovation in 3D point cloud technology within the AEC field, creating greater value for its client's projects. As LiDAR technology continues to advance and reshape the AEC industry, BasicAI stands ready to support professionals in navigating these changes, helping them effectively utilize 3D point cloud data to enhance their future projects.
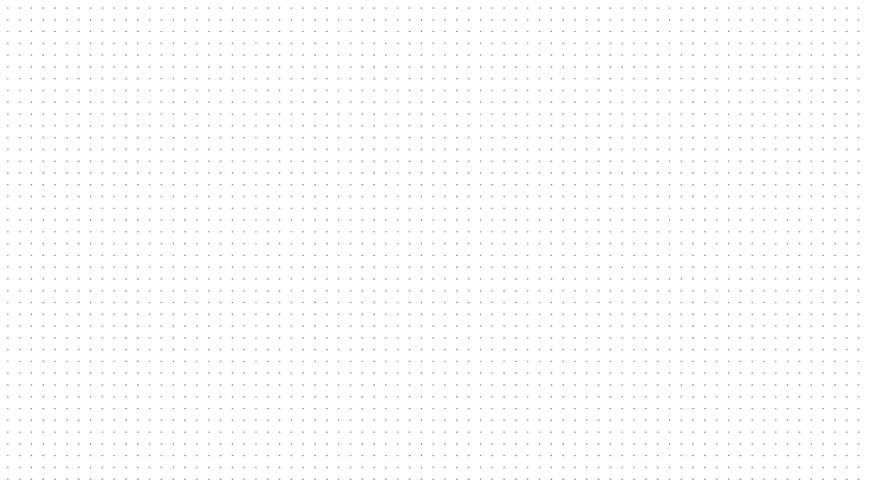