We have discontinued our cloud-based data annotation platform since Oct 31st. Contact us for private deployment options.
When it comes to 3D mapping projects, the quality of your training data can make or break your success. With a vast array of data annotation tools available, it's essential to choose the one that will give your project the competitive advantage it deserves. Enter point cloud annotation – the unsung hero of the 3D mapping world.
How To Choose A Suitable Annotation Tool For A CV Project?
When selecting a suitable annotation tool for your computer vision project, consider the tool's compatibility with your data format and required annotation types, its efficiency and user-friendliness to streamline the annotation process, and its scalability to handle your project's size. Additionally, ensure that the chosen tool can seamlessly integrate with your existing machine learning pipeline and other tools you may be using.
In this blog post, we'll explore the world of point cloud annotation and its critical role in 3D mapping. We'll cover its unique characteristics, recent technological advancements, a real-world use case, and future trends. By the end, you'll have a solid understanding of how point cloud annotation can elevate your 3D mapping projects to new heights.
Must-know Elements to Grasp
Point Cloud
Point clouds are datasets composed of numerous points in space, each containing position information, used to represent the three-dimensional shape of object surfaces or spaces. This data is widely applied in fields such as 3D modeling, surveying, and autonomous driving to accurately capture and reproduce the physical characteristics of the real world.
Point cloud technology is continuously being optimized and improved. The Point Transformer V3 (PTv3), proposed by Zhaochong An et al., emphasizes simplicity and efficiency to enhance scalability in 3D point cloud processing. It has achieved state-of-the-art results in various indoor and outdoor 3D perception tasks, significantly improving processing speed and memory efficiency compared to its predecessor, PTv2. Additionally, the COSeg model, proposed by Xiaoyang Wu et al., optimizes the association between support and query, improving generalization ability to new categories and achieving state-of-the-art results in the FS-PCS field.
Point Cloud Annotation
Point cloud annotation is the process of labeling and categorizing individual points or groups of points within a point cloud dataset. This annotation process is crucial for training machine learning models to accurately interpret and understand 3D data, enabling applications such as object detection, semantic segmentation, and scene understanding in fields like autonomous driving, robotics, and 3D mapping.
Is Point Cloud Annotation Equal to Lidar Annotation?
Point cloud annotation is the process of labeling features within any 3D point data, while LiDAR annotation specifically refers to this process for point clouds generated by LiDAR technology. So, while LiDAR annotation is a type of point cloud annotation, they are not equal because point cloud annotation can involve data from other sources as well. In addition, lidar point cloud annotation is a combination of the above two types of annotation.
🌟 You may be interested in How to Annotate 3D LiDAR Point Cloud 82 Times Faster with Higher Accuracy.
3D Mapping
3D mapping involves creating digital representations of the physical world by capturing, analyzing, and visualizing 3D data. This technology is crucial for autonomous driving and finds applications in various other fields, such as urban planning, construction, virtual reality, and gaming. In autonomous vehicles, 3D maps are generated using a combination of LiDAR, cameras, GPS, and other sensors to produce layered, informative models of the world that the vehicle's systems can interpret and act upon.
Exploring the Role of Point Cloud Annotation in 3D Mapping Workflow
In 3D mapping, point clouds play a crucial role by providing the raw data needed to create detailed three-dimensional models of physical spaces and objects. Below is how point clouds work in 3D mapping:
Data Acquisition: Point clouds are generated using 3D scanning technologies like LiDAR, structured light scanning, or photogrammetry.
Data Representation: Each point in a point cloud has coordinates (x, y, z) and may include additional data such as intensity and color information.
Point Cloud Processing: Raw point clouds are filtered, segmented, and classified to isolate and organize relevant points.
3D Model Reconstruction: Processed point cloud data is used to reconstruct a 3D model of the environment by creating a mesh or using complex modeling techniques.
Mapping and Localization: In autonomous driving, 3D maps created from point clouds serve as a reference for vehicles to localize themselves and navigate accordingly.

A Specific Use Case: HERE HD Live Map
HERE HD Live Map is an advanced mapping service developed by HERE Technologies, which is essential for autonomous vehicle navigation. It uses point cloud data collected from specialized mapping vehicles equipped with LiDAR sensors. This data undergoes a detailed annotation process, where features such as road lanes, traffic signs, and other critical infrastructure elements are identified and labeled. The annotated point cloud is then integrated into a multi-layered HD map that provides precise road geometries, lane information, and a localization layer to assist autonomous vehicles in real-time decision-making. This high-definition map is continuously updated to reflect the changing road conditions, making it a dynamic tool for the safe operation of self-driving cars.
Source: https://www.here.com/platform/HD-live-map
Where Will It Go in 2024?
Point Cloud Technology
The future of point cloud technology is exciting. As sensor technologies like LiDAR advance and costs decrease, point cloud data acquisition will become more prevalent and efficient. For example, Tesla's new Autopilot system uses advanced LiDAR sensors for precise environment perception and modeling, greatly improving the safety and reliability of autonomous driving. In industry, point cloud modeling and analysis can optimize production processes and improve quality, as demonstrated by Mitsubishi Electric's digital transformation of their factories.
Advancements in AI will lead to breakthroughs in point cloud data processing and analysis. Deep learning algorithms for tasks like semantic segmentation and object detection will become more mature and widely applied. NVIDIA's cuDNN library supports efficient point cloud data processing, greatly accelerating the training of point cloud deep learning models. Waymo's point cloud segmentation algorithm enables real-time detection and tracking of vehicles and pedestrians, providing crucial data for autonomous driving decisions. The integration of point cloud technology with other cutting-edge technologies like AR and robotics will spawn even more innovative applications, such as Microsoft's HoloLens 2 using point clouds for realistic holographic interactions. In summary, point cloud technology has boundless potential and will undoubtedly become a key technology driving human society's progress.
Point Cloud Annotation in 3D Mapping
Firstly, high-resolution and real-time mapping will become a reality. For example, Leica Geosystems' latest BLK2GO handheld imaging laser scanner can capture point cloud data at a speed of 420,000 points per second and generate high-resolution 3D models in real-time. These real-time, dynamic 3D maps will reflect instant changes in the environment, providing up-to-date digital representations for various industries. Secondly, semantic information will be deeply integrated with 3D maps. For instance, the Mapp platform developed by the British startup SenSat can automatically analyze point cloud data, extract semantic attributes of elements such as roads and buildings, and create "intelligent" 3D maps. These semantically rich maps will support advanced queries and analysis, offering more comprehensive support for decision-making.
Furthermore, multi-source data fusion will become a significant trend in 3D mapping. For example, the Cesium platform by the American technology company Analytical Graphics Inc. can seamlessly integrate point cloud data with satellite imagery, Geographic Information System (GIS) data, and other multi-source data to create complete and accurate 3D digital worlds. Collaborative mapping and crowdsourcing will also play a crucial role in the future. Open-source platforms like OpenStreetMap allow volunteers worldwide to jointly create and maintain global 3D map data through crowdsourcing. This participatory mapping process will empower the public to shape the expression of their digital environment.
Lastly, annotated point cloud data and 3D maps will find wide applications across various domains. In the field of urban planning, Singapore's "Virtual Singapore" project utilizes detailed 3D city models for urban design, planning, and management. In the domain of disaster response, the U.S. Federal Emergency Management Agency (FEMA) uses point cloud data collected by drones to quickly assess the damage to buildings after disasters, guiding rescue and reconstruction efforts.
Point Cloud Annotation: Empowering High-Precision 3D Mapping
With the rapid development of 3D mapping technology, high-quality point cloud data annotation has become crucial for improving mapping accuracy and efficiency. Converting raw point cloud data into structured data that can be used to train machine learning models is a complex and time-consuming task, requiring a high level of expertise and technical skills.
At our company, a team of professionals is ready to provide you with efficient and precise point cloud annotation services. Our team members not only have extensive experience in the mapping industry but have also acquired the necessary skills for point cloud annotation through specially designed training courses. Whether you use LiDAR point clouds, oblique photogrammetry data, or other types of 3D mapping data, we can provide annotation solutions tailored to your unique needs.
🌟 You may be interested in Should You Tackle Data Annotation In-House or Outsource Data Labeling Work?
Through our point cloud annotation services, you can obtain high-quality structured data, thereby enhancing the accuracy and reliability of your mapping results. This annotated data can be used not only for traditional mapping applications, such as terrain modeling and urban planning but also to support the development of emerging fields like autonomous driving and smart cities.
If you are interested in our point cloud annotation services, please feel free to contact us for more details. We look forward to collaborating with you to drive innovation and application in 3D mapping technology.
Reference
[1] Wu, Xiaoyang et al. “Point Transformer V3: Simpler, Faster, Stronger.” ArXiv abs/2312.10035 (2023): n. Pag.
[2] Kong, Lingdong et al. “Rethinking Range View Representation for LiDAR Segmentation.” 2023 IEEE/CVF International Conference on Computer Vision (ICCV) (2023): 228-240.
Read Next
AI In Agriculture | 7 Applications of AI in Agriculture [2024 Updated]
AI In Autonomous Driving| Data Annotation for Autonomous Driving: How Labeled Data Helps Cars Drive Itself?
AI In Construction | 7 Useful Applications of AI in Construction
AI In Education| The Influence of Artificial Intelligence in Education and the Impact of Data Annotation Services
AI In Healthcare| Harnessing AI in Healthcare: The Role of BasicAI's Data Annotation
AI In Insurance | How AI Helps in the Insurance Industry [4 Applications]
AI In Logistics | The Future Driven by Artificial Intelligence and Data Annotation
AI In Manufacturing| Data Annotation for Manufacturing AI and Industrial Automation Model Training
AI In Retail | AI and the Retail of the Future: BasicAI's Data Annotation Makes it Possible
AI In Security| Driving Security Forward: The Role of AI and Data Annotation in Modern Security Solutions
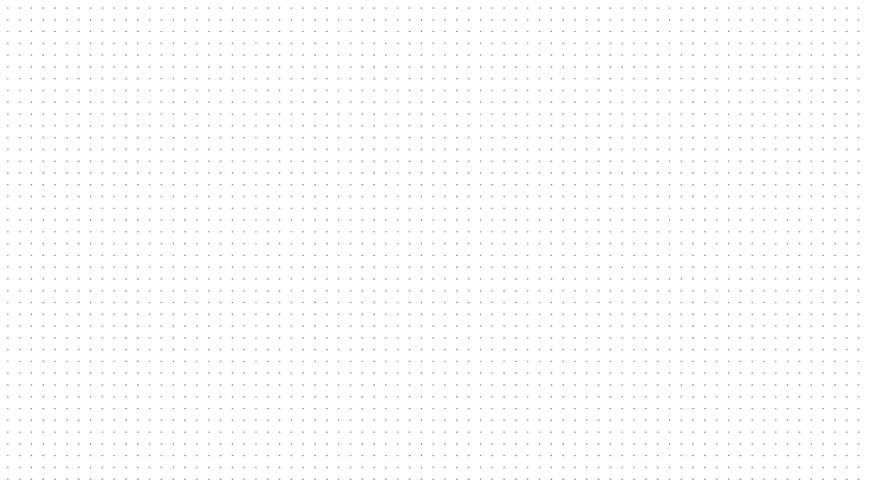