We have discontinued our cloud-based data annotation platform since Oct 31st. Contact us for private deployment options.
Artificial Intelligence is playing an increasingly pivotal role in the security sector, applied to areas including cybersecurity, premises surveillance, supply chain logistics, and beyond. The global market for AI in security is anticipated to reach a staggering $37 billion by 2026, according to MarketsandMarkets. However, the effectiveness of AI largely hinges on the quality of training data, a factor causing an estimated 92% of AI projects to fail (Forbes).
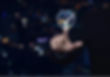
To mitigate this challenge, companies like BasicAI offer crucial support. With over seven years of experience in annotating a broad range of security data, BasicAI brings invaluable expertise to the table. Our services empower security companies to build high-accuracy AI systems, capable of countering a multitude of threats. By enhancing the quality of training data, BasicAI plays a crucial role in harnessing the full potential of AI, thereby significantly boosting overall security measures.
The Growing Role of AI in Security
In recent years, the adoption of Artificial Intelligence in the security field has marked a significant evolution in how security measures are implemented and managed. AI's ability to learn from and make sense of large volumes of data makes it a powerful tool for enhancing security across multiple domains. The applications of AI in the field of security are diverse and far-reaching:
Biometric Authentication: AI can effectively process and analyze biometric data such as fingerprints, facial recognition, and voice recognition, leading to more secure and personalized access to devices and systems. According to a study by Spiceworks, 62% of companies will use biometric authentication by 2023.
Surveillance Monitoring: AI-powered surveillance systems can analyze video footage in real-time, detecting unusual activities and alerting security personnel. This drastically reduces response times to security incidents, which can be vital in preventing damage or loss. A recent IBM study found that AI could help businesses identify a breach within 201 days, 52 days faster than manual processes.
Insider Threat Detection: AI can identify anomalous activities that may indicate potential insider threats by learning and understanding typical user behaviors. This includes unusual data access patterns or suspicious network activity.
Fraud Prevention: AI can analyze transactions in real-time, detecting patterns indicative of fraudulent activity, which can significantly reduce financial losses and protect users' sensitive information. A study by Juniper Research predicts that by 2023, AI will save businesses $7 billion annually in fraud detection.
These applications underscore the growing role of AI in security, marking both exciting opportunities and significant challenges.
The Dual Nature of AI in Security: Benefits and Challenges
In the realm of security, Artificial Intelligence brings a host of benefits to the table, acting as a formidable force in predicting, detecting, and reacting to threats.
Proactive Risk Identification: AI's capacity to analyze trends and patterns in security data allows it to forecast potential security threats. Consequently, organizations can proactively address vulnerabilities before they're exploited, enhancing their security posture.
Processing Massive Data: With the ability to quickly process and analyze vast amounts of data, AI can identify threats with a speed and accuracy far surpassing human capabilities. This results in faster response times and more effective threat mitigation.
Complementing Human Analysts: Far from replacing human analysts, AI complements them, taking over routine tasks and thereby allowing analysts to focus on complex security issues that require human intuition and experience.

However, the integration of AI within security frameworks is not without its hurdles:
Large Training Datasets: AI systems thrive on high-quality data for training, which can prove challenging in security contexts where data is often sensitive or hard to come by.
Reliable Labeling: The effectiveness of AI hinges on accurately labeled training data. Improperly labeled data can lead to inaccurate results, posing potential security risks.
Strong Evaluation Metrics: Assessing the efficacy of an AI security system can be complex. Clear, robust evaluation metrics are crucial to ensure the AI system performs as expected and enhances security.
While the growing role of AI in security presents challenges, it also offers immense potential for improving security measures and protecting against increasingly sophisticated threats, if implemented and evaluated carefully. Therefore, having a reliable partner to assist in the preparation and management of AI training data can be invaluable.
BasicAI's Security Annotation Services: A Key Asset in Your AI Journey
Recognizing the importance of high-quality training data and the challenges involved in its preparation, BasicAI offers specialized security annotation services to optimize the performance of your AI models for security applications. Here are the key benefits of our services:
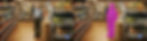
Improved Accuracy of AI Through High-Quality Labeled Data: At BasicAI, we understand that the success of any AI model hinges on the quality of its training data. We provide high-quality data annotation services, ensuring your AI models are trained on accurately labeled data. This significantly improves their ability to accurately detect security threats and anomalies, mitigating the risk of false positives and negatives.
Faster Model Development by Leveraging Our Annotation Expertise: The development of an AI model is a lengthy process, with data annotation often being one of the most labor-intensive parts. By utilizing BasicAI's expertise in data annotation, you can expedite the development of your AI models. Our experienced annotators are proficient in handling a wide variety of security data, enabling us to deliver accurately annotated data in a timely manner and speed up your model development process.
Allowing Security Teams to Focus on Core Tasks by Outsourcing Annotation: Data annotation, while crucial, is a resource-intensive task. By outsourcing this process to BasicAI, your security teams can concentrate on their primary responsibilities, such as threat analysis and strategy development. This not only boosts your team's productivity but also allows them to focus on strategic tasks that can not be automated.
BasicAI's security annotation services provide a strategic edge in the development and implementation of AI in your security systems. By ensuring high-quality annotated data, accelerating your model development, and freeing up your security team's time, we enhance the efficacy of your AI security solutions.
Security Data Annotation Use Cases
Data annotation plays a pivotal role in training AI models, especially in the field of security. Through the application of accurate labels on diverse types of security data, AI models can learn to identify patterns indicative of threats or anomalies. Here are several use cases where data annotation significantly contributes to AI's capabilities in security.
Annotating Surveillance Video for Activity Recognition and Threat Identification
Surveillance videos offer a rich source of data for AI. However, raw video footage is unstructured data that AI models find challenging to interpret. Data annotation comes into play here, turning raw video into a structured format that AI can understand.
Video annotation involves labeling different elements within the footage, such as people, objects, and activities. For instance, an annotator might label a person leaving a bag unattended in a public place as "suspicious activity." By learning from these labels, an AI model can eventually recognize similar actions in new footage, enhancing its ability to identify potential threats in real-time.
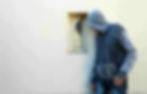
Labeling Access Logs to Detect Compromised Credentials and Insider Risks
According to a report by Cybersecurity Insiders, 63% of organizations say data loss prevention is their top priority for insider threat management, and AI can significantly aid in this.
Access logs record who has accessed a particular system and when providing crucial data that can be used to detect security breaches. For example, unexpected access from an unfamiliar location or at an unusual time may indicate compromised credentials.
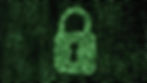
By annotating this data - labeling instances of normal and abnormal access patterns - AI models can be trained to automatically detect signs of compromised credentials or insider threats. This can significantly enhance an organization's ability to respond swiftly to security incidents.
Annotating Shipping Data to Identify Supply Chain Anomalies
Supply chains generate extensive data, from manufacturing timelines to shipping details. Anomalies within this data can indicate potential issues, such as delays, fraud, or theft. A 2020 study by the University of Surrey found that AI could reduce supply chain disruptions by up to 50%.
Data annotation involves labeling different elements in the supply chain data, such as shipping locations and quantities. For example, the location of a ship could be labeled with GPS coordinates as it moves from port to port. An anomalous event, like a ship taking an unusual route or deviating significantly from its planned course, could be labeled as a "potential delay" or "possible piracy threat." An AI model trained on this annotated data can then monitor new ship location data for similar anomalies, alerting relevant parties to potential issues in the supply chain.

Data annotation plays a crucial role in leveraging AI for security. By accurately labeling diverse types of security data, AI models can be trained to identify, predict, and respond effectively to various security threats and anomalies.
Takeaways
In conclusion, the landscape of security is being significantly reshaped by the advent of AI. However, the success of AI in this field hinges on the quality of training data. This crucial aspect of AI development, often overlooked, is where companies like BasicAI come to the forefront. Our extensive experience in data annotation, coupled with a deep understanding of the security sector, allows us to provide a vital service. By enhancing the quality of data for AI training, BasicAI helps security companies develop AI systems that are not just accurate but robust in countering a diverse range of security threats. As we move forward, the role of data annotation in AI will continue to grow, and partnerships with companies like BasicAI will be essential in navigating the future of security.
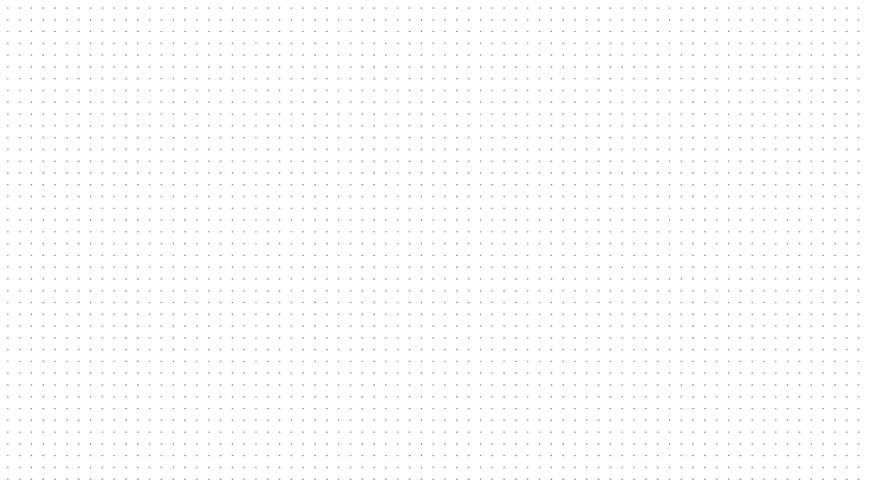