We have discontinued our cloud-based data annotation platform since Oct 31st, 2024. Contact us for private deployment options.
Imagine a world where machines can see, interpret, and understand images just like humans. According to Gartner, by 2025, 75% of enterprise-generated data will be created and processed outside traditional data centers, with images and videos forming a substantial part of it. In this scenario, computer vision, an AI technology that enables computers to understand and label images, is set to play a pivotal role.
At the heart of this transformation is a technique known as keypoint annotation. It's a crucial process that marks the specific points on an object within an image or video frame, providing critical inputs for machine learning models. As per MarketsandMarkets, the image recognition market is projected to grow from USD 26.2 billion in 2020 to USD 53.0 billion by 2025. Keypoint annotation, being a significant part of this process, is instrumental in driving this growth.
In this guide, we'll delve into the world of keypoint annotation, discussing its applications, challenges, and how BasicAI, a leading player in data annotation tools, is facilitating its usage across various industries. Whether you're an AI expert, a budding researcher, or someone intrigued by the potential of AI, this exploration promises to be insightful and exciting.
Keypoint Annotation Explained
Keypoint annotation is a crucial process in the field of computer vision that involves marking specific points or 'keypoints' on an object within an image or video frame. Unlike bounding boxes and polygons to draw rectangles or outline the objects' shapes, these key points capture unique and important features of the object, providing critical information about its structure and position. The process forms the backbone of machine learning models' ability to 'see' and interpret visual data, making it an essential component of any computer vision task. This kind of annotation can be used to model anything from the human body's structure for pose estimation to specific facial features for facial recognition systems.
The significance of keypoint annotation is evident in its wide-ranging applications across various industries. In healthcare, it's used to annotate medical images, aiding in disease diagnosis and surgical planning. In security and surveillance, it powers facial recognition and behavior analysis systems. The retail industry leverages it for virtual try-on systems and touchless interfaces, while in manufacturing, it helps in automating quality control. Thus, keypoint annotation is more than just marking points on an image; it's a crucial step in training AI systems to understand and interact with the world in a meaningful way, revolutionizing numerous sectors in the process.
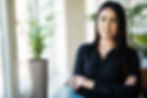
Exploring the Diverse Range of Data Types for Keypoint Annotation
Keypoint annotation, an integral aspect of computer vision, is applicable to an expansive variety of data types. Its versatility extends beyond static images, encompassing video sequences, 3D data, medical imaging, and even aerial and thermal images. This wide applicability underscores its significant role in numerous fields and applications.
Image Data: This is the most common type of data where keypoint annotation is applied. It includes marking specific points of interest in static images, such as facial features in facial recognition systems or object points in object detection tasks.
Video Data: Keypoint annotation in video involves marking points of interest across multiple frames. This is often used in motion analysis, behavior tracking, and activity recognition. For instance, in sports analytics, keypoint annotation can help track the movement of players over time.
3D Data: Keypoint annotation can also be applied to 3D data like point clouds generated by LiDAR or 3D models. For instance, in autonomous driving, keypoints on 3D point clouds can help identify and understand the shape and position of various objects in the environment.
Keypoint annotation is a fundamental concept in computer vision. In computer vision tasks, algorithms use these annotations to learn and make predictions about new, unseen data. Therefore, accurate keypoint annotation is crucial for the success of these applications, underpinning advancements in areas.
On the other hand, BasicAI is a prominent player in the field providing robust data annotation tools and services. The company is committed to accelerating the development of artificial intelligence by offering high-quality, human-annotated data for machine learning models. BasicAI's platform is equipped with a diverse set of annotation tools, including keypoint annotation, which enables users to precisely mark points of interest on images or video frames. In addition, BasicAI provides a range of services that include data collection, data annotation, and model evaluation, offering end-to-end solutions for businesses and researchers in their AI development journey.
Pros and Cons of Keypoint Annotation
Keypoint annotation has its advantages and disadvantages. While it offers detailed information, flexibility, and efficiency, it also presents challenges such as demanding accuracy requirements, subjectivity, scalability issues, and complexities in ontology creation. However, platforms like BasicAI have risen to the task, offering solutions designed to address these concerns and streamline the annotation process.
Pros
Detailed Information: Keypoint annotation provides detailed information about the structure and shape of objects, which can lead to more accurate and nuanced models.
Flexibility: It can be used across a wide range of applications, from facial recognition to pose estimation and object tracking.
Efficiency: Compared to segmenting an entire object, marking a few key points can often be faster while still providing valuable information.
Cons
Accuracy Requirements: Keypoint annotation demands a high degree of accuracy and precision. Each point must be placed correctly to ensure the resulting model's efficacy.
Subjectivity: Different annotators might interpret 'key' points on an object differently, leading to inconsistencies in the data.
Scalability: Annotating keypoints on large datasets can be overwhelming and time-consuming, posing challenges in terms of scalability.
Ontology Creation: The process of creating a well-defined ontology for keypoints can be complex, especially for those new to this aspect of data annotation.
Challenges Solved by BasicAI
Recognizing these challenges in keypoint annotation, BasicAI has designed its platform to provide solutions that simplify the process. To address the need for accuracy, BasicAI's user-friendly and precise interface assists annotators in marking keypoints accurately and quickly.
BasicAI also mitigates the subjectivity issue by providing clear instructions and guidance for annotators, along with implementing quality checks to ensure consistency in the annotation process.
Lastly, the challenge of creating a comprehensive ontology is addressed by offering resources and guidance that help users understand and defining their ontologies effectively. In this way, BasicAI ensures a streamlined and efficient keypoint annotation process, aiding in the development of high-quality datasets for AI projects.

Harnessing Keypoint Annotation: Real-World Applications
Keypoint annotation is a critical component in many applications within the domain of computer vision. Here are several use cases where keypoint annotation plays a vital role:
Human Pose Estimation
In fields such as sports analytics, healthcare, and animation, understanding the pose of a human body is incredibly valuable. Keypoint annotations can be used to mark specific points on a human body, such as joints, to create a model of human poses.
Facial Recognition and Emotion Detection
Keypoint annotation can be used to identify specific points on a face, such as the corners of the eyes, the tip of the nose, the corners of the mouth, etc. These key points can then be used for facial recognition or to detect emotions based on changes in these key points.

Object Detection and Recognition
Keypoint annotations can help to detect and recognize objects within images or video frames. By marking key points on an object, machine learning models can learn to recognize these objects in other images or videos.
Autonomous Vehicles
In the field of autonomous driving, key point annotation can help identify and understand objects on the road. For instance, key points can be used to outline pedestrians, other vehicles, traffic signs, and more, enabling the autonomous system to better understand its surroundings.
Gesture Recognition
In virtual reality or augmented reality environments, understanding human gestures is key to creating interactive experiences. Keypoint annotation can be used to model these gestures, allowing the system to respond to human actions.
Medical Imaging
In healthcare, key point annotation is used to detect and outline specific points of interest within medical images such as CT scans or MRIs. For instance, key points can be used to identify the boundaries of a tumor or other medical conditions.
Best practice with BasicAI Approaches
Step 1: Upload the Dataset
To begin, you'll need to upload your dataset to the BasicAI Cloud* platform. If you don't already have an account, you'll need to create one. The platform supports various data sources including local data, URLs, as well as cloud storage, both BasicAI's storage and your personal cloud storage. Select the images or videos that you want to annotate and upload them. It's important to assign unique names to each of your files for easy identification and efficient data management, as these names will be visible in the annotation interface.

Step 2: Define Ontology
The next step involves creating an ontology for your data. This is a crucial process as it provides a class or category for your key points. To create an ontology, you'll need to add basic information such as the name, color, and tool type. Once you've filled in these details, you can complete the ontology, effectively providing a structured framework for your annotation process.

Step 3: Annotate the Object with Key point
This is the most critical step in the data annotation process. Navigate to the annotation platform interface and select the keypoint annotation tool. You can then proceed to label the required objects in your images or videos. Ensure you choose the corresponding label for each keypoint based on the ontology you defined earlier. This process involves marking specific points on an object that captures essential features, providing valuable input for your machine learning model.

Step 4: Export the Annotated Data
After completing the annotation process, you can export your data. BasicAI makes this process straightforward - simply click the 'Export' button. You'll receive accurately labeled data, ready to be used for training your machine learning model. This data will include the images or videos you uploaded, along with the keypoint annotations you created, providing a rich dataset for your AI projects.

Leveraging Keypoint Annotation with BasicAI
Keypoint annotation, due to its critical role in computer vision, is seeing increasing adoption across diverse industries. As an indispensable tool in the AI toolkit, it enables machines to interpret and understand the visual world, driving innovation and efficiency. BasicAI, as a leader in this space, offers robust and versatile tools for keypoint annotation, accelerating the development of high-quality datasets for AI projects.
In conclusion, keypoint annotation, far from being merely a tool for marking points on an image, is a powerful enabler in the quest to make machines 'see' and interact with the world. With BasicAI's robust tools and services, the journey towards achieving this nuanced understanding is made significantly smoother.
* To further enhance data security, we discontinue the Cloud version of our data annotation platform since 31st October 2024. Please contact us for a customized private deployment plan that meets your data annotation goals while prioritizing data security.
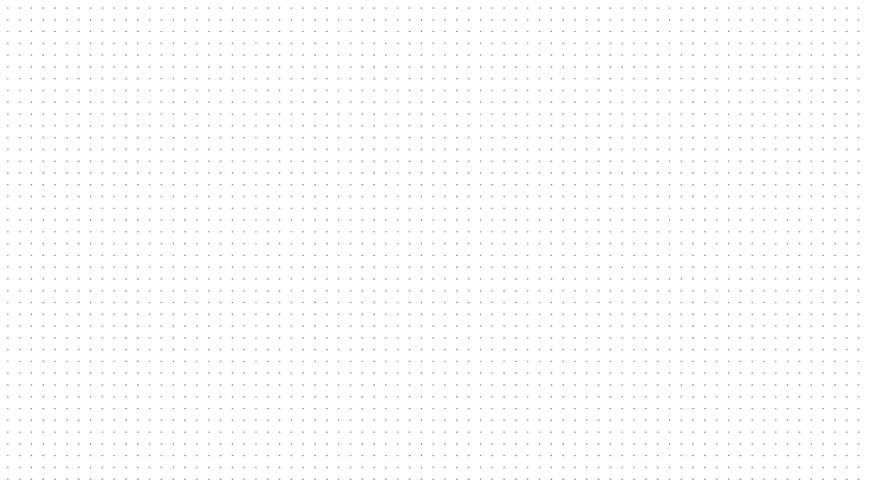